EPS Colloquium – Yongji Wang, NYU
Unraveling the Physical Properties of Antarctica Ice: A Data-Driven Discovery
Mass loss from the Antarctic Ice Sheet is one of the major contributors to sea level rise. Ice shelves—the floating extensions of the ice sheet—play a critical role in regulating ice discharge into the ocean, mitigating sea-level rise. However, the rheology of glacial ice (its deformation under stress) at ice-shelf scales (hundreds of kilometers) remains an open question in glaciology for over 60 years, limiting our ability to predict Antarctica’s future evolution. In this talk, I will show how we integrate remote observations, fundamental mechanics and physics-informed machine learning to unravel the rheology of Antarctic ice shelves. Our findings reveal substantial differences in rheology between compression and extension zones. In compression zones (near grounding lines), glacial ice follows a grain size–sensitive composite rheology, aligning with laboratory experiments on polycrystalline ice. In contrast, ice in extension zones (especially near calving fronts) exhibits anisotropic properties. We inferred the first ice shelf–wide maps of anisotropic viscosity from the data, identifying suture zones that inhibit rift propagation. The discovery of composite rheology and anisotropic viscosity dictates the fate of ice shelves—from their initial discharge from the grounded ice sheet to eventual collapse into the ocean—making them essential for predicting future Antarctic ice loss.
To be added to the EPS colloquium mailing list, please contact Caroline Carr at carolinecarr@fas.harvard.edu
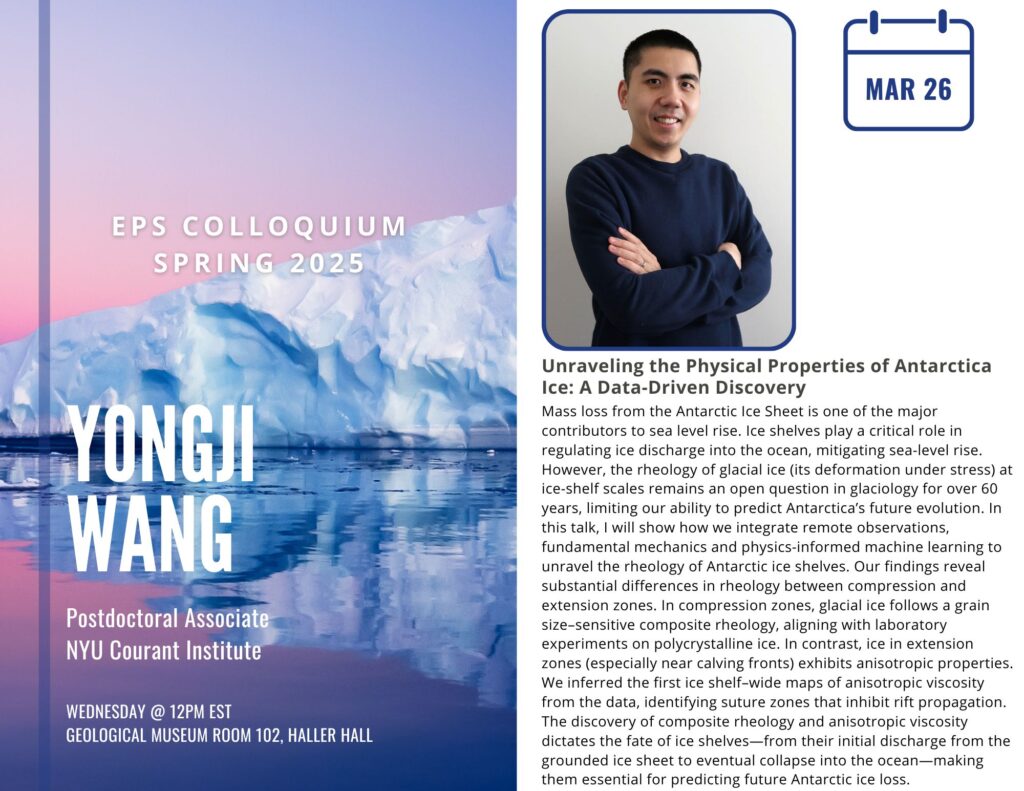
Yongji Wang (he/him) is currently a postdoc at Courant institute of Mathematical Sciences at New York University (NYU) and also a visiting postdoc at Stanford University. His research interests lie in continuum mechanics, geophysics, and scientific machine learning. He has rich experience in developing theoretical and numerical techniques for elucidating complex physical processes in nature and environment. He received his PhD in Civil and Environmental Engineering from Massachusetts Institute of Technology (MIT) and his master’s degree in applied Mathematics from the University of Cambridge with a Distinction. His current research focuses on developing high-precision deep-learning techniques for various scientific problem, from uncovering hidden physical properties of Antarctica ice shelves to finding self-similar blow-up solutions for nonlinear partial differential equations (PDE).